Yield 101: A/B Tests
For as much as I mock adtech content, there’s actually an enormous amount of high-quality shit written. There are great explainer series from Digiday and Jounce Media. Adexchanger and Rob Beeler’s daily emails are must-reads. MSG’s quarterly updates are ESSENTIAL to understanding what has or will happen. Ari Paparo, Kerry Flynn, and Paul Bannister are must-follows on twitter. And then just make sure you read everything Josh Sternberg, Lara O’Reilly, Ronan Shields, and Craig Silverman write.
Yet despite all of the fantastic content being created daily, you know what I have never once read? A single actionable bit of yield advice. Yield is core to what we do but it feels like something left for “nerds” with “spreadsheets” to “figure out” as they go.
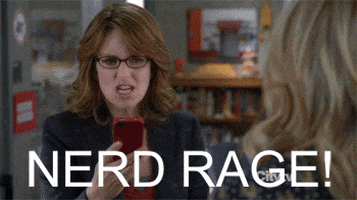
What ends up happening then is every yield person starts their career blind and whether by dumb luck, purposeful study, or helpful elders they start to acquire a black book of yield tricks. The really good ideas get shared in hushed tones, with close friends only. Taken too far some people can mistakenly believe their value is their book of tricks and not the method they use to get those tricks. Which, given these uncertain times, feels incredibly stupid.
So I’m going to start sprinkling some of the yield tricks I’ve learned over these years. Whenever possible I’ll give screenshots or examples but no matter what my goal is for everyone to be able to take what I write and literally use them that day. So with that in mind let me introduce my very first Yield 101:
How To A/B Test (without bugging your dev team)
The two core tenants to good yield practice are testing and reporting. If you can’t apply the scientific method to your yield then you have little hope in getting the most out of it.
The problem most publisher rev ops teams run into is that A/B testing requires dev work. Which means at most you can do a handful of tests a quarter. When you should actually be running multiple tests a month. So how do you bridge this gap?
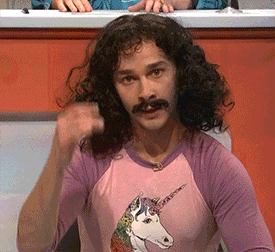
The magic of javascript. Ask your dev team to build a key-value that applies a random number (1-100) to every single ad slot on the page. If you want to see it in the wild just add “?googfc” to the end of any article on The Atlantic (also, subscribe!). You’ll see it there, clear as day, a randomized 1-100 value with the key value of “abt” (a/b test).
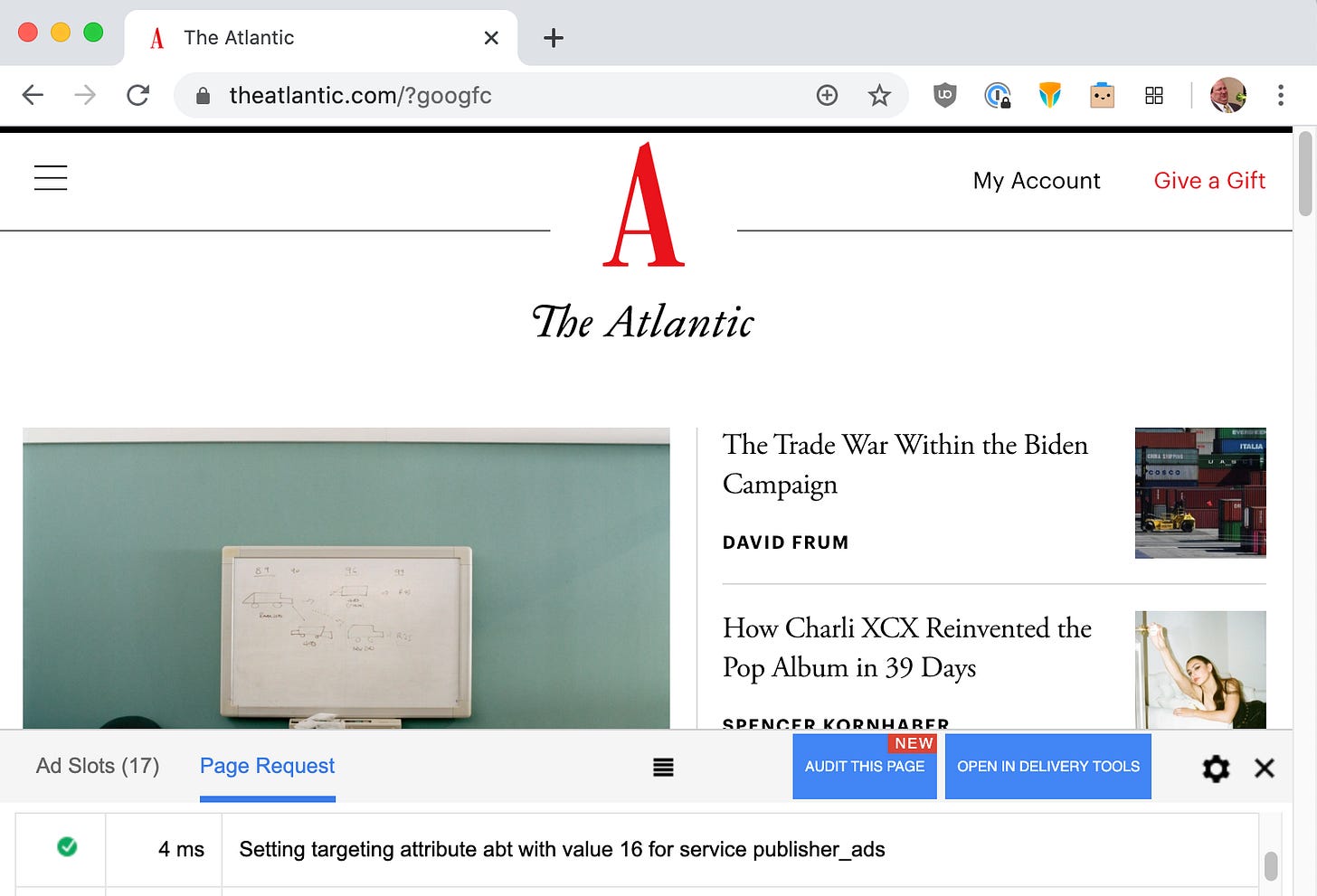
With this one simple move, I can run any number of A/B tests on a single-digit percent of my inventory without ever needing my dev team. Tests such as:
Easy Tests
Would working with [x] vendor make us more money? Let’s run a test on 10% of our inventory to see.
What if we only run a vendor on mobile/desktop? Do we make more $$?
Testing frequency caps for high impact units and determining their effect on revenue/impressions.
Intermediate Tests
Run tests on your lazyload settings
This requires dev work but now you can have your dev team test multiple different lazyload settings on small percentages of your inventory and you can compare the impression loss/viewability gain to your control.
Run tests on prebid timeout settings
Same as above but now you can test different timeouts. Maybe even different timeouts for different devices. A slightly more advanced version would allow your dev team to also compare page load speeds across those tests.
Advanced Tests
Run kill tests with each vendor you work with to quantify their revenue uplift.
Exclude that vendor from a specific abt value. Compare that to your control (a key-value you never apply a test to). If you make more on the kill value than the control then you know that vendor isn’t providing lift.
Do session-based abt values as well
We have the standard abt. But we also have a value that gives a random 1-100 to every ad unit for a user’s whole session. This allows us to run tests on the effects of different ad density experiences. Or different positions of native/ad/recirc units. Honestly, your creativity is your limit.
Limitations
This won’t work off your O&O. So anyone with a sizeable amount of AMP, Apple News, or FBiA traffic won’t be able to run these same tests on those platforms.
It’s not perfect. It’s hard to do a true control with this method (you can’t exclude adx cleanly with abt values).
For those who aren’t getting log-level data from DFP you’ll need to be smart with how you do reporting. Since key-value reporting in DFP is very limited. Make sure you take the time to plot this all out before you start your experiments.
For the more advanced stuff, it’s important that you’re also able to capture page analytics data so make sure those ABT values are passed to GA.
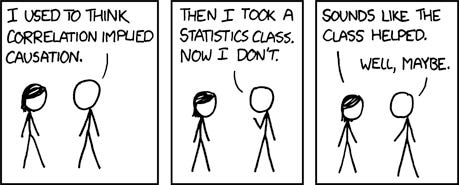
If you haven’t already surmised, getting a fundamental understanding of statistics is almost a prerequisite to running and evaluating proper A/B tests. There are plenty of places online to learn this stuff and it doesn’t take too long. Do yourself a favor. Read up.
Next. Make sure your excel skills are up to snuff. At a minimum, you should be able to handle pivot tables, concat, sumif, ifs, and vlookups or index/matches.
Ok, that’s all for me today. I look forward to hearing from all the greybeard yield people on how dumb everything I just said was. The rest of you I hope I gave you a few ideas. Feel free to send me any other a/b tests we should be running.
Shameless Self Promotion
Check out Erik and I’s newest episode of What Happens in Adtech. This week we talked to Scott Messer about SPO, DPO, and PADs among other things. Scott also showed off his extensive zoom background collection.
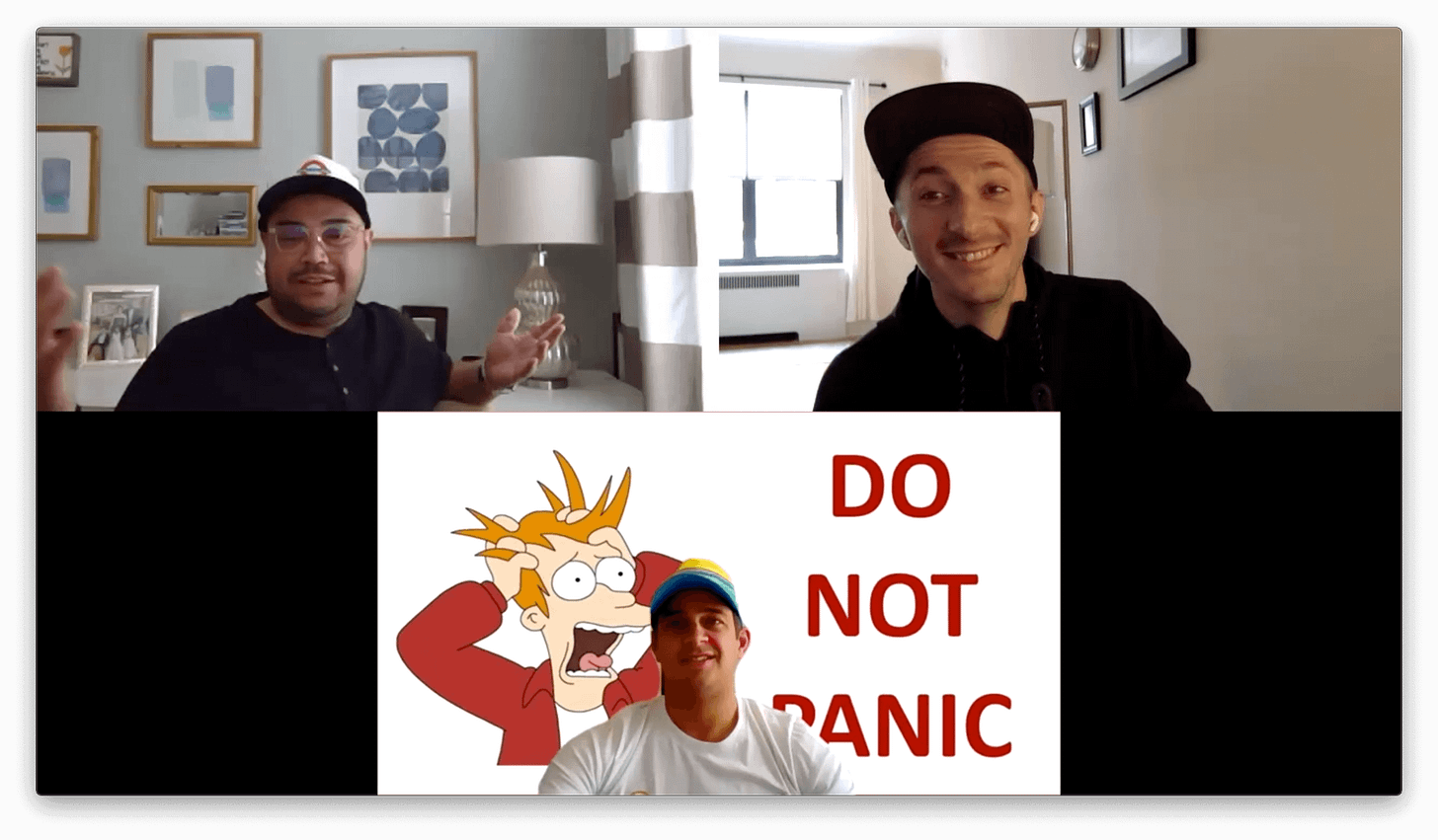
We also offer an audio-only version of WHIAT on Spotify and iTunes. Subscribe!
If you like the words I wrote above please share it with other smart people:
Ok, that’s all for me. Have a great day, or don’t. I’m not the boss of you!